Single View Metrology in the Wild
- 1UC San Diego
- 2Adobe
Overview
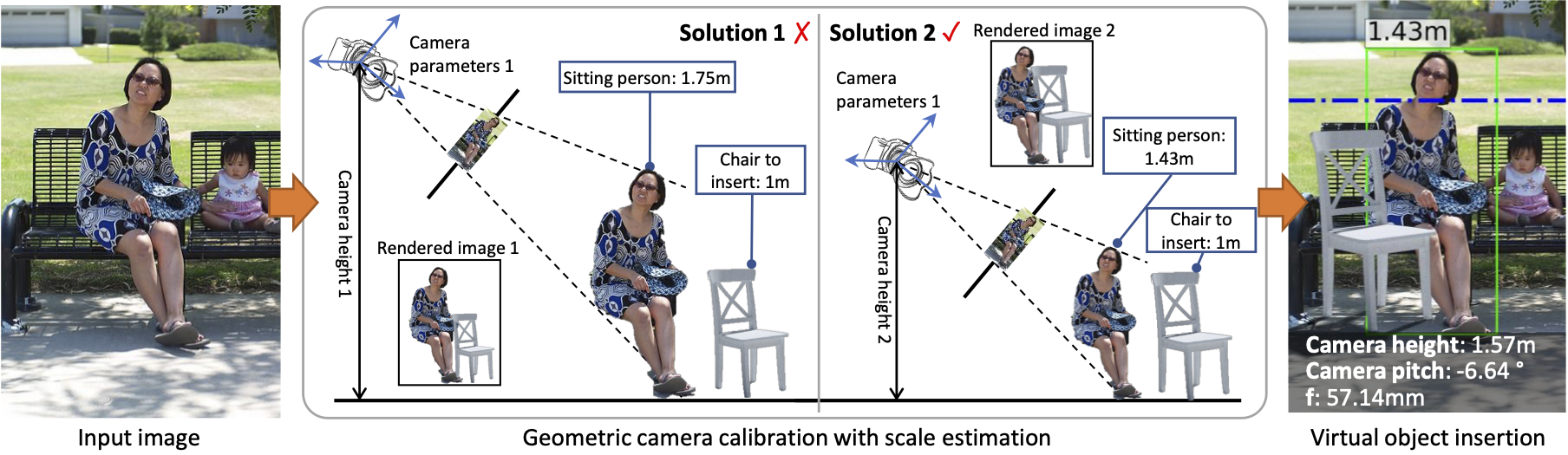
Most 3D reconstruction methods may only recover scene properties up to a global scale ambiguity. We present a novel approach to single view metrology that can recover the absolute scale of a scene represented by 3D heights of objects or camera height above the ground as well as camera parameters of orientation and field of view, using just a monocular image acquired in unconstrained condition. Our method relies on data-driven priors learned by a deep network specifically designed to imbibe weakly supervised constraints from the interplay of the unknown camera with 3D entities such as object heights, through estimation of bounding box projections. We leverage categorical priors for objects such as humans or cars that commonly occur in natural images, as references for scale estimation. We demonstrate state-of-the-art qualitative and quantitative results on several datasets as well as applications including virtual object insertion. Furthermore, the perceptual quality of our outputs is validated by a user study.
Highlights
- A state-of-the-art Single View Metrology method for images in the wild that performs geometric camera calibration with absolute scale—-horizon, field-of-view, and 3D camera height—from a monocular image.
- A weakly supervised approach to train the above method with only 2D bounding box annotations by using an in-network image formation model.
- Application to scale-consistent object insertion in unconstrained images.
- A new dataset and benchmark for single image scale estimation in the wild.
- All code and trained models are to be publicly available.
Long Video
Download
All code and datasets are to be released on Github.
Acknowledgments
Parts of this work were done when Rui Zhu was an intern at Adobe Research.
The website template was borrowed from Zhengqin Li and Michaël Gharbi.